Forecasting and Demand Planning in 2025 – A Comprehensive Guide
- Mar 6
- 7 min read
The Future of Forecasting: Smarter, Faster, and More Accurate
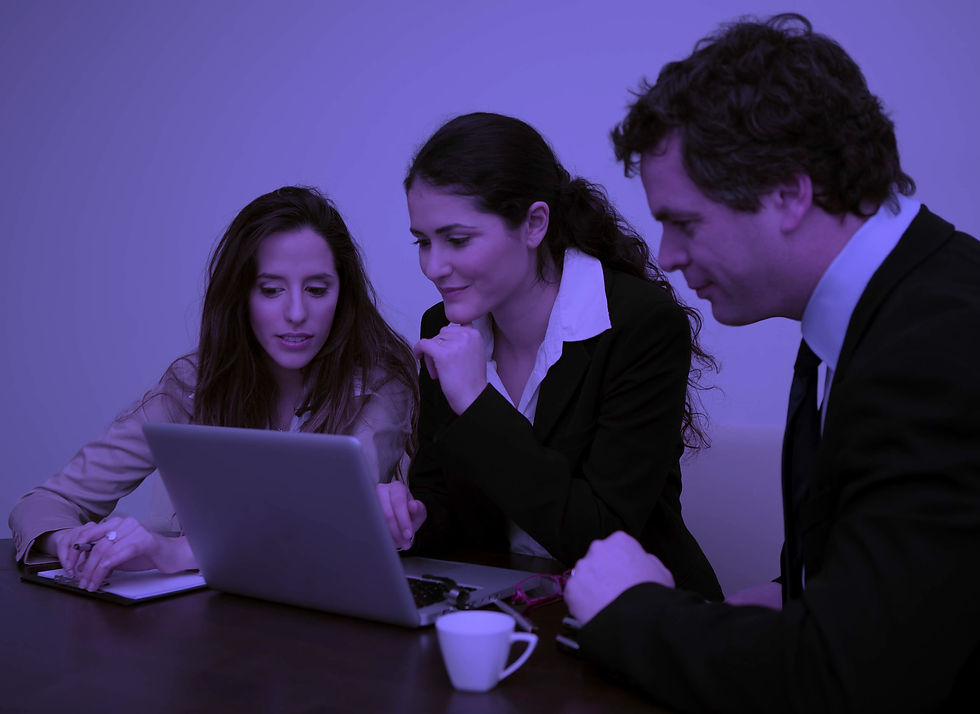
Imagine knowing exactly what your customers will need before they do. In 2025, forecasting and demand planning are no longer just about educated guesses—they’re powered by AI-driven insights, real-time data, and automation. Businesses that fail to adopt these advanced methods risk inefficiencies, stock shortages, and lost revenue.
So, how do you stay ahead?
This guide will walk you through the latest trends, best practices, and must-have tools for mastering forecasting and demand planning in 2025. Whether you're a supply chain manager, business strategist, or operations expert, this is your roadmap to accuracy, efficiency, and growth.
Why Forecasting and Demand Planning Matter More Than Ever
With global supply chain disruptions, evolving consumer behaviors, and economic uncertainties, businesses can’t afford to rely on outdated forecasting methods. Accurate demand planning is the key to:
Reducing stock outs & overstock by keeping inventory levels optimized.
Maximizing profits by avoiding waste and unnecessary holding costs.
Enhancing customer satisfaction by ensuring products are available when needed.
Improving operational efficiency by streamlining production and logistics.
Traditional vs. Modern Forecasting Approaches
Method | Accuracy | Scalability | Adaptability |
Historical Data-Based | Medium | Low | Low |
Rule-Based Forecasting | Medium | Medium | Medium |
AI-Driven & Predictive Analytics | High | High | High |
In the next section, we’ll explore how forecasting is evolving and what technologies are shaping the future of demand planning in 2025.
The Shift to AI-Driven Forecasting
In 2025, artificial intelligence (AI) and machine learning (ML) are redefining demand forecasting. Traditional forecasting methods often rely on historical data and fixed models, but AI-powered forecasting adapts in real time by analyzing market conditions, consumer behavior, and economic trends.
Why AI-Driven Forecasting is Essential
Real-time adjustments – AI models continuously update forecasts based on live data.
Pattern recognition – Identifies hidden trends humans might miss.
Scenario modeling – Predicts the impact of market changes, disruptions, and demand fluctuations based on computed scenarios.
Automation – Reduces the need for manual intervention, freeing up valuable human time for strategic planning.
Example:
A retail company using AI-driven forecasting notices a sudden spike in demand for eco-friendly packaging due to a social media trend. Instead of waiting for traditional reports, AI identifies the pattern early, prompting the company to ramp up production before competitors catch on.
The Role of Predictive Analytics in Demand Planning
Predictive analytics combines big data, AI, and statistical models to improve forecasting accuracy. Unlike historical forecasting, predictive models adjust to:
Economic shifts such as inflation rates, interest rate changes, and global trade disruptions.
Consumer behavior trends like changes in preferences, shopping habits, and seasonal demand.
Supply chain variables including but not limited to weather conditions, geopolitical risks, and production constraints.
Key Predictive Analytics Models for 2025:
Model | Purpose |
Time-Series Forecasting | Uses historical data trends to predict future demand |
Regression Analysis | Identifies relationships between demand and external factors |
Machine Learning Models | Continuously improve accuracy through real-time data analysis |
Simulation & Scenario Planning | Tests different market conditions to assess risk |
Case Study: A logistics company implemented predictive analytics to anticipate port delays. By analyzing real-time shipping data and weather patterns, they adjusted delivery schedules, avoiding costly disruptions in their supply chain.
The Rise of Digital Twins in Forecasting
A digital twin is a virtual replica of a supply chain, market, or business operation that updates in real time using live data. Companies are leveraging digital twins for:
Simulating demand fluctuations before they happen.
Optimizing production schedules based on predictive data.
Reducing waste and inefficiencies by testing multiple demand scenarios.
Example: An electronics manufacturer created a digital twin of its global supply chain. When a key supplier faced delays, the digital model simulated alternative sourcing strategies, preventing stock outs and lost revenue.
In the next section, we'll break down best practices for implementing modern forecasting techniques and how businesses can transition from outdated models to data-driven demand planning.
Best Practices for Transitioning to Data-Driven Demand Planning
Shifting from traditional demand planning to a data-driven, AI-powered forecasting approach can seem overwhelming. However, companies that successfully transition benefit from higher accuracy, reduced waste, and improved profitability. Here’s how to make the switch efficiently:
1. Centralize Data Sources
One of the biggest obstacles to accurate forecasting is fragmented data spread across multiple departments and systems. Centralizing data allows businesses to:
Ensure consistency across all forecasting models.
Improve real-time collaboration between supply chain, sales, and finance teams.
Reduce errors from manual data transfers.
Tip: Invest in an ERP system with AI-driven analytics to bring all data together in one place.
2. Implement AI-Driven Forecasting Gradually
While AI-powered forecasting delivers outstanding results, implementing it all at once can disrupt workflows. Instead, businesses should:
Start with a pilot program for a specific product line or region.
Compare AI-generated forecasts against traditional methods to measure improvements.
Gradually expand AI-driven forecasting across other business units.
Example: A fashion retailer tested AI-based demand forecasting on seasonal collections. The AI model outperformed human predictions by 15.39%, reducing excess inventory costs and increasing profit margins.
3. Incorporate Real-Time Data Feeds
Static forecasting models fail in fast-changing markets. To stay ahead, integrate real-time data from sources like:
Customer purchasing trends (e.g., online sales, in-store foot traffic, social media sentiment).
Global supply chain shifts (e.g., port delays, factory shutdowns, raw material shortages).
Macroeconomic factors (e.g., inflation rates, currency fluctuations, geopolitical risks).
Case Study: A food distributor used real-time weather data to adjust perishable goods inventory in different regions. This reduced waste by 12% and increased on-time order fulfillment.
Enhancing Forecasting Accuracy with AI-Powered Training
Even with advanced forecasting tools, human decision-makers still play a crucial role. Upskilling teams in AI and predictive analytics ensures they can:
Interpret AI-generated insights accurately.
Make informed decisions based on real-time forecasts.
Fine-tune automation settings to match business needs.
Where to Get Started?
CohesivApp’s AI Bootcamp offers hands-on training for businesses looking to integrate AI-powered forecasting into their operations. Participants learn:
How to transition from manual forecasting to AI-driven models.
Best practices for data integration and real-time analytics.
Strategies to increase accuracy and responsiveness in demand planning.
🎯 Want to stay ahead of the curve? Join the AI Bootcamp today and future-proof your forecasting strategy!
In the next section, we'll explore common forecasting mistakes and how to avoid them, helping businesses refine their demand planning processes for 2025 and beyond.
Common Forecasting Mistakes and How to Avoid Them
Even with advanced forecasting tools, businesses still make critical mistakes that lead to inventory imbalances, lost revenue, and operational inefficiencies. Here are the most common demand planning mistakes and strategies to avoid them in 2025.
1. Relying Solely on Historical Data
The Mistake: Many businesses still depend on past sales trends to predict future demand. However, consumer behaviors, market conditions, and supply chain factors are evolving rapidly, making historical data less reliable.
How to Avoid It:
Incorporate real-time data feeds into forecasting models.
Use AI-driven predictive analytics to adjust demand forecasts dynamically.
Factor in external variables like weather, economic trends, and competitor activity.
Example: A consumer electronics brand relying only on past sales underestimated demand for a new product launch. By incorporating social media trends and pre-order insights, they could have adjusted production in real time to meet demand.
2. Ignoring Market Volatility and Supply Chain Disruptions
The Mistake: Businesses assume supply chains will remain stable, leading to stock shortages or excess inventory when disruptions occur.
How to Avoid It:
Use scenario planning tools to model different supply chain risk situations.
Adopt real-time inventory tracking to quickly adjust stock levels.
Implement automated reordering systems that respond to changing demand.
Case Study: A global apparel retailer using CohesivApp’s demand planning tools modeled multiple supply chain scenarios. When a key fabric supplier faced delays, the AI model recommended an alternative supplier, preventing stockouts and lost sales.
3. Overcomplicating Forecasting Models
The Mistake: Some companies introduce overly complex forecasting models that require extensive manual adjustments, leading to delays and errors.
How to Avoid It:
Start with simplified AI-driven models before scaling up.
Focus on key demand indicators rather than tracking too many variables.
Automate forecasting adjustments with machine learning algorithms.
Example: A manufacturer manually adjusting 100+ demand variables switched to AI-based forecasting, cutting forecasting errors by 40% and improving supply chain responsiveness.
The Role of AI Training in Avoiding Forecasting Mistakes
Why Training Your Team Matters
Even with best-in-class AI forecasting tools, human oversight is still essential. Teams need to understand, interpret, and act on AI-driven forecasts effectively.
This is where CohesivApp’s AI Bootcamp comes in!
✔ Learn how to transition from outdated forecasting methods to AI-driven demand planning. ✔ Master real-time data analysis and predictive modeling. ✔ Avoid common forecasting mistakes with hands-on AI training.
🎯 Take control of your demand planning today! Enroll in the AI Bootcamp and give your team the competitive edge.
In the final section, we’ll discuss the future of forecasting and how businesses can stay ahead in 2025 and beyond.
The Future of Forecasting: Staying Ahead Beyond 2025
The future of forecasting and demand planning is evolving rapidly. Businesses that want to stay ahead must embrace next-gen technologies, real-time data, and AI-driven automation. Looking beyond 2025, hyper-accurate forecasting will become a competitive necessity rather than an advantage.
Key Trends Shaping the Future of Forecasting
1️⃣ AI-Powered Autonomous Demand Planning
Forecasting will move from assisting decision-makers to making real-time autonomous decisions.
AI models will continuously self-improve based on historical performance, live market data, and predictive analytics.
2️⃣ Real-Time Demand Sensing
Businesses will use instant data collection from social media, IoT sensors, and online transactions to adjust forecasts in real time.
Demand shifts will no longer be predicted monthly or weekly but hourly and daily, enabling more agile supply chains.
3️⃣ Blockchain for Transparent Supply Chains
Secure, decentralized forecasting will allow businesses to share live demand data with suppliers and distributors in real time.
Improved trust and accuracy will help prevent bullwhip effects in supply chains.
4️⃣ Digital Twins for Forecasting Optimization
Companies will build digital twins of supply chains, allowing them to simulate demand fluctuations before they happen.
These virtual models will optimize production schedules, inventory levels, and transportation logistics instantly.
How Businesses Can Stay Competitive
Adopt AI-driven forecasting tools now to build a strong data foundation for future automation.
Invest in predictive analytics training for teams to interpret and act on AI-generated forecasts.
Upgrade ERP and demand planning systems to support real-time adjustments and automated decision-making.
The Final Step: Training Your Team for the Future
Even the best AI-powered forecasting tools are only as effective as the people using them. Bridging the knowledge gap between traditional forecasting and autonomous AI-driven demand planning is critical.
Future-proof your business with CohesivApp’s AI Bootcamp!
✔ Learn how to integrate AI into demand planning.
✔ Master real-time forecasting techniques.
✔ Gain hands-on experience with predictive analytics models.
🎯 Get ahead of the competition—Enroll today and take forecasting into the future!
Final Thoughts
The future of forecasting belongs to companies that embrace automation, real-time data, and AI-powered decision-making. By staying ahead of industry trends, adopting cutting-edge technology, and investing in AI training, businesses can ensure long-term growth, resilience, and success in an increasingly unpredictable market.
Is your company ready for the next era of demand planning? Start today and lead the future of forecasting!
Opmerkingen